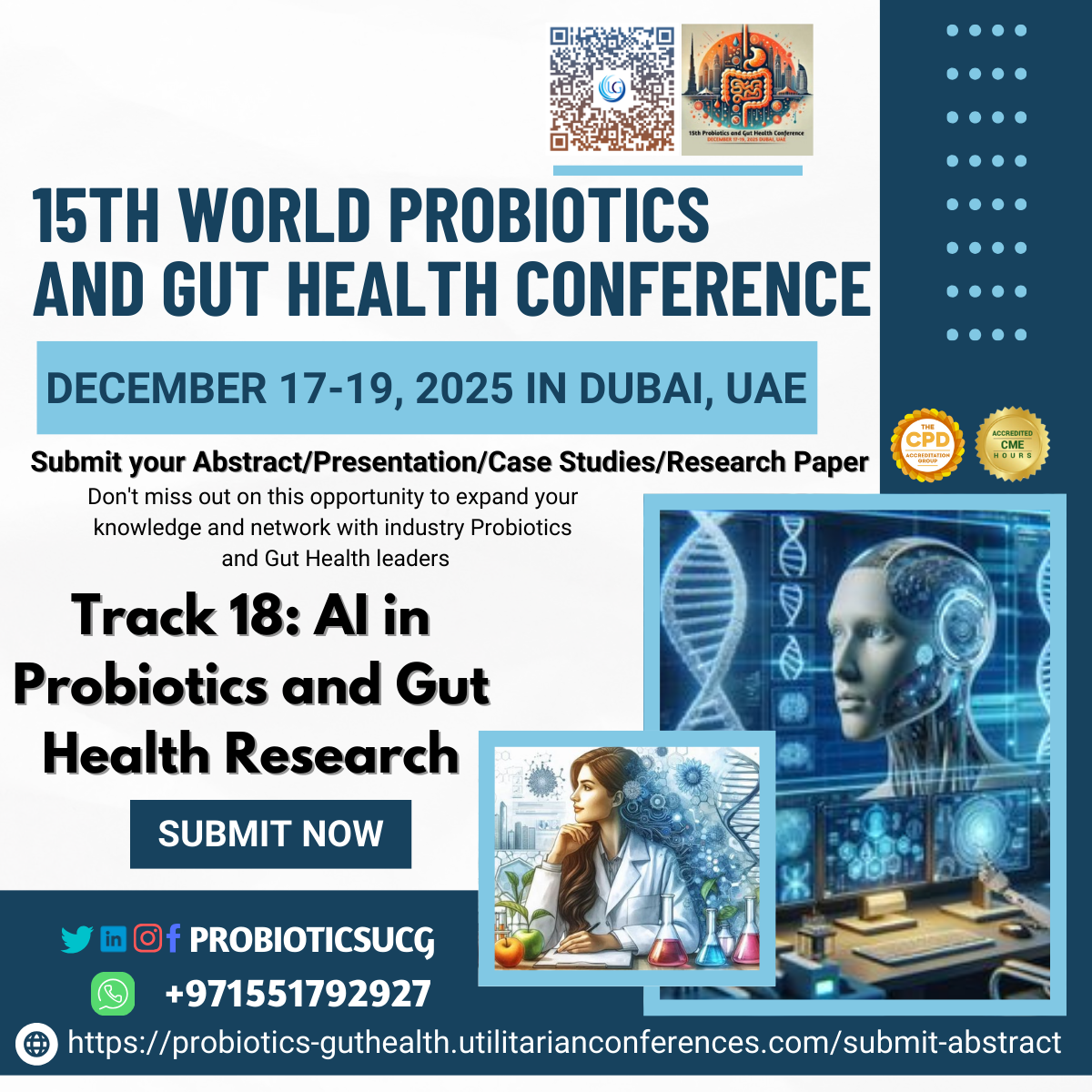
Probiotics are live microorganisms that provide health benefits when consumed in adequate...
Gut health refers to the balance and functionality of the digestive system,...
AI in Probiotics & Gut
Health Research
Artificial
Intelligence (AI) is revolutionizing many fields, and probiotics and gut health research are no
exception. AI technologies, such as machine learning (ML), natural language
processing (NLP), and deep learning, are enhancing our understanding of the gut
microbiome, the mechanisms through which probiotics affect health, and how
personalized interventions can improve outcomes.
AI's
application in probiotics and gut health research is still evolving but holds
immense promise in several areas, from microbiome analysis to the development
of personalized probiotics.
Applications of AI in
Probiotics & Gut Health Research
1.
Microbiome Profiling and
Data Analysis
High-Throughput Sequencing: AI plays a key role in
analyzing microbiome data generated by sequencing technologies (e.g., 16S rRNA
sequencing, metagenomics, etc.). These techniques generate large datasets that
need to be processed and interpreted efficiently. AI algorithms help in
identifying the types of microorganisms present in the gut, their functional
roles, and how they interact with one another and with the host.
Pattern Recognition: Machine learning models
can identify patterns and correlations within the microbiome data, such as
those linking specific bacteria to health outcomes like IBD, obesity, diabetes, or mental health. This helps
researchers uncover novel biomarkers and mechanisms of disease, improving our
understanding of how the microbiome influences overall health.
2.
Identification of Probiotic
Strains
Predicting Probiotic Efficacy: AI can be used to predict
which probiotic strains will have specific health benefits. By analyzing large
datasets of clinical trial outcomes and microbiome profiles, machine learning
models can identify strains that have the potential to treat or prevent certain
health conditions (e.g., gut dysbiosis, inflammatory bowel disease).
Optimizing Strain Selection: AI models can analyze the
genetic, metabolic, and functional properties of probiotic strains to identify
the best candidates for therapeutic use. This can speed up the process of
developing new probiotics and ensure they are tailored to address specific
health issues.
3.
Personalized Probiotics
Microbiome-Based Personalization: One of the most exciting
applications of AI in probiotics is the development of personalized probiotics. AI
algorithms can integrate data from an individual’s gut microbiome, genetics,
lifestyle, diet, and health conditions to recommend specific probiotic strains
or combinations that are most likely to improve their health.
Tailoring Probiotic Interventions: Personalized approaches
to probiotics are being researched to provide more effective treatments. AI can
help predict how an individual will respond to a particular probiotic and guide
researchers in designing more effective and targeted interventions for
conditions like gut inflammation,
autoimmune disorders, and metabolic diseases.
4.
Predicting Health Outcomes
and Disease Risks
Gut Health Risk Prediction: AI models can integrate
microbiome data with clinical health records to predict an individual's risk of
developing diseases linked to gut health, such as irritable bowel syndrome (IBS),
Crohn’s disease, obesity, or cardiovascular diseases. These
predictive models can help identify individuals who might benefit most from
probiotic interventions before symptoms appear.
Clinical Trials: AI-driven approaches can
streamline clinical trial designs by identifying patient populations most
likely to benefit from probiotics, improving recruitment, and helping to
predict how patients will respond to treatment. AI can also assist in
monitoring clinical trial outcomes by analyzing complex data in real time.
5.
Enhancing Probiotic
Formulation
Formulation Optimization: The development of new
probiotic products involves not only selecting the right strains but also
determining the best delivery mechanisms (capsules, powders, fermented foods).
AI can optimize the formulation process
by predicting the most effective combination of strains, doses, and delivery
methods based on various factors such as the individual's gut health status and
age.
Stability and Efficacy Predictions: AI can model how
probiotics behave under different environmental conditions, such as changes in
temperature, humidity, or the acidic environment of the stomach. This helps in
formulating probiotics that maintain their effectiveness and stability over
time, ensuring that they reach the gut alive and active.
6.
Improving Gut Health
Diagnostics
Disease Diagnosis: AI can improve the
accuracy of gut health diagnostics by analyzing complex datasets from clinical
tests, medical imaging, and microbiome sequencing. For instance, AI can detect
early signs of diseases like IBD or Celiac disease by analyzing gut
microbiota and its response to probiotic interventions.
Early Detection of Dysbiosis: AI can be used to
identify early signs of dysbiosis
(microbial imbalance in the gut), which is linked to a variety of conditions
such as digestive disorders, immune dysfunction, and even mood disorders. Early
intervention with probiotics can prevent the development of these conditions.
7.
AI in Gut-Brain Axis
Research
Gut-Brain Communication: AI is helping to uncover
the complex communication between the gut microbiota and the brain (the gut-brain axis). By analyzing
neuroimaging data, microbiome data, and behavioral outcomes, AI can identify
how probiotics influence mental health conditions like anxiety, depression, and stress.
Predicting Mental Health Outcomes: AI models can predict the
potential effects of specific probiotics on the brain by considering an
individual's gut microbiome composition, lifestyle factors, and genetic
predispositions.
8.
AI-Driven Literature Mining
and Knowledge Discovery
Natural Language Processing (NLP): AI-powered NLP tools are
being used to analyze large amounts of existing research on probiotics and gut
health. These tools can extract valuable insights from published papers,
clinical trial data, and medical records, helping researchers stay up to date with
the latest findings.
Knowledge Graphs and Databases: AI helps build
comprehensive knowledge graphs that connect different probiotic strains, their
health benefits, and associated diseases. These can be used by researchers to
identify new relationships between probiotics and gut health conditions.
Challenges and Limitations
While
AI is making significant strides in probiotics and gut health research, several
challenges remain:
Data Quality and Standardization:
The
effectiveness of AI models is highly dependent on the quality of the data they
are trained on. Microbiome data is often noisy, incomplete, and lacks
standardization, which can limit the accuracy and reproducibility of AI-driven
insights.
Complexity of the Gut Microbiome:
The
gut microbiome is highly dynamic and influenced by a multitude of factors,
including diet, environment, genetics, and lifestyle. AI models need to account
for this complexity when predicting probiotic efficacy or gut health outcomes.
Ethical and Privacy Concerns:
The
use of personal health data, including microbiome data, raises concerns about
privacy and data security. Ethical considerations must be addressed, especially
when implementing AI in personalized medicine.
Regulatory Challenges:
The
use of AI in probiotics and gut health research must be aligned with healthcare
regulations, especially in the context of personalized medicine and clinical
trials. Regulatory bodies will need to keep pace with the rapid development of
AI technologies in this field.
Conclusion
AI
is increasingly playing a transformative role in probiotics and gut health
research by improving our understanding of the microbiome, enhancing the
precision of probiotic interventions, and enabling personalized approaches to
health. With the ability to analyze large datasets, identify new probiotic
strains, and predict health outcomes, AI is accelerating the development of
targeted therapies for gut-related diseases and improving overall health
management. However, as research continues, addressing challenges related to
data quality, privacy, and regulatory frameworks will be essential to fully
harness the potential of AI in this field.